1. 问题分类
针对问题的分类
- Factoid: who is the president of USA?
- Simple Question. One that can be answered with single evidence. E.g. Who wrote the book of Beijing Folding
- Multi-hop Question. Requires with many facts
- Aggregate Question. Requires with many facts and calculation. E.g. what is the longest Olympic opening before Beijing 2008.
- Descriptive: what are characteristics of the new Mac Pro
- Procedural: how to install windows 10
- Calculation: how many Chinese won Turing awards
- Causal: why is it dark at night
- Opinion: how do you think about Trump?
2. 基于不同数据形式的问答
2.1 传统问答系统基本架构
Factoid Question Answering System
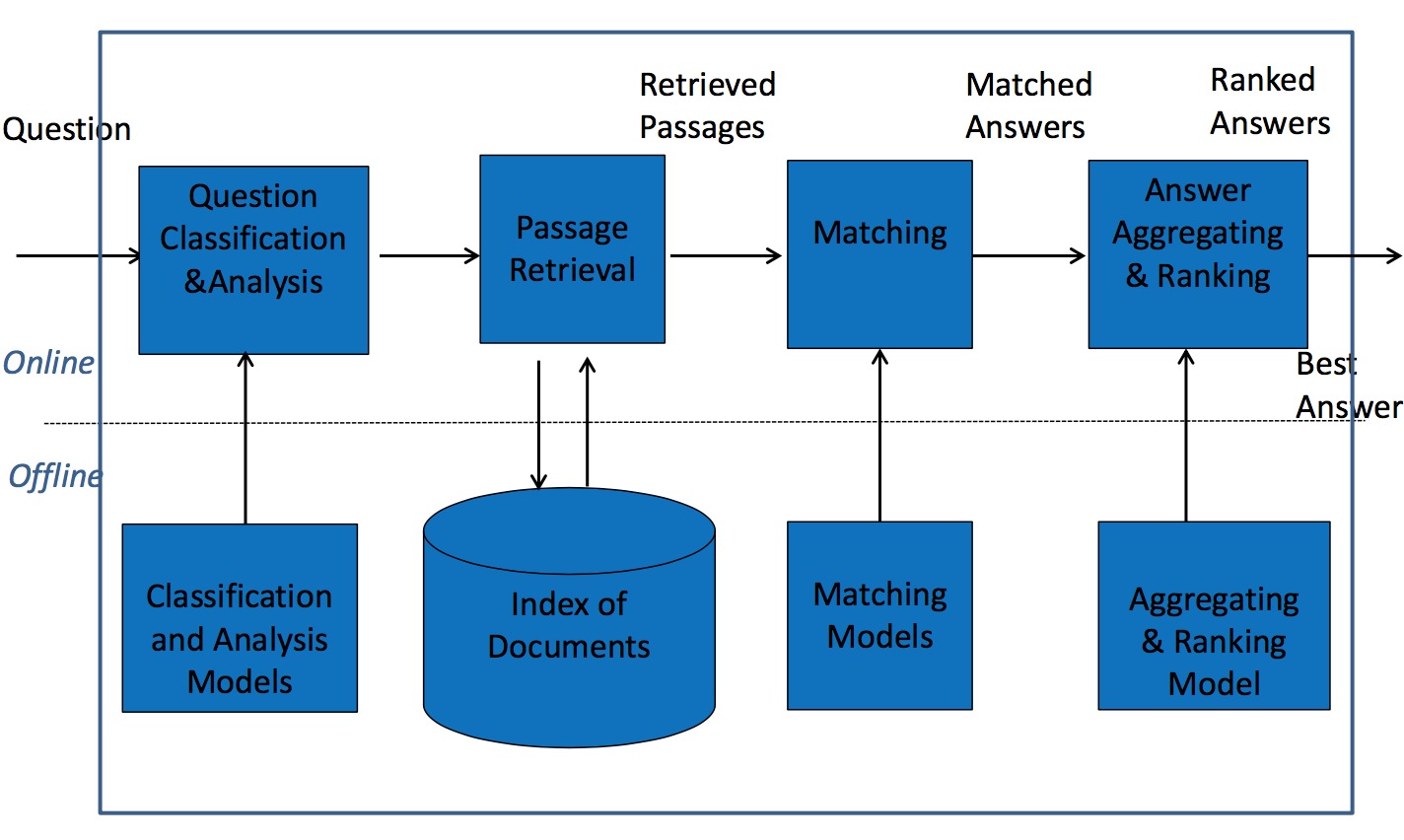
Community Question Answering System

2.2 基于结构化数据的问答(知识图谱、数据库)
2.2.1 主要问题
如何匹配自然语言问题与结构化的知识图谱triple,包括问句句式变换、实体别名处理等。
- Embedding,将问题、triple都映射成向量进行匹配。
如何根据问题和匹配到的triple生成自然语言答案。
- 基于RNN的生成模型,类似seq2seq的decoding过程。
2.2.2 相关模型
CFO:首先通过sequence labelling方法找出focus短语,通过TransE的方法把知识图谱中的实体与关系都转化成Embedding。然后通过Stacked bi-directional GRU生成问题的Embedding,分别计算句子的Embedding与句子中出现的实体/关系的距离来最终生成答案。

KBQA:基本思路是利用问题模板来明确用户意图。首先识别问题中的实体,然后根据问题和实体寻找可能对应的模板,通过模板推测出其对应的用户意图,最终转化成知识图谱查询。

GenQA:首先将query和知识图谱中的triple转化成embedding,通过query word生成triple候选,通过CNN的方法计算query与triple候选的相似度并选择最匹配的triple,最后基于生成模型生成最终的答案。
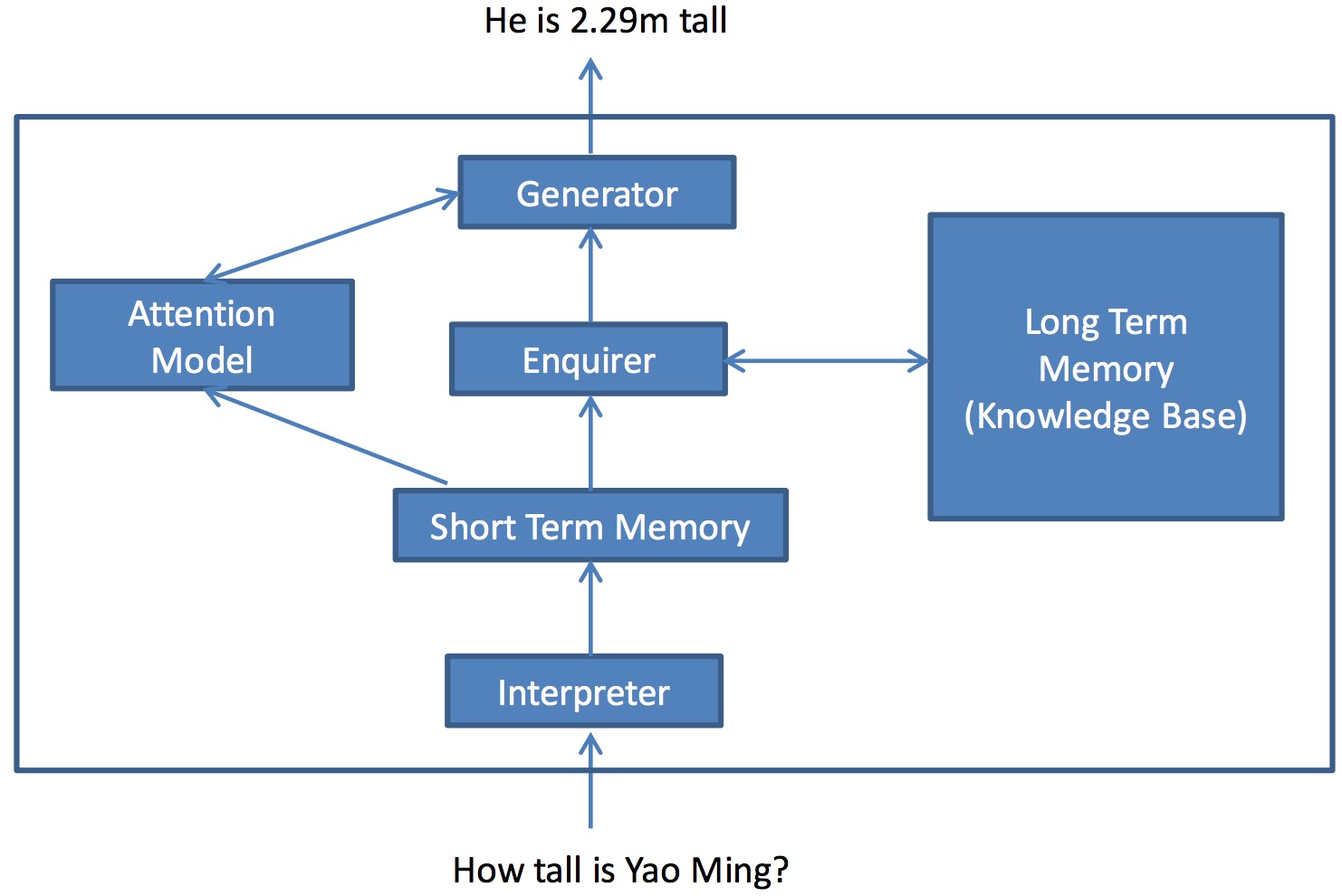
QA from Relational Database:基于关系型数据库的问答系统,首先将数据库中的entry转化成table embedding,将query也转化成embedding,然后基于Memory Network类似的思路对逐步将query转化成关系型数据库查询。

2.3 基于FAQ的问答
2.3.1 主要问题
如何从半结构化/无结构化的数据中挖掘FAQ。
- QnA Miner,采用机器与人工相结合的半自动方法来解决。
如何匹配问题与答案。
- 经典的Ranking问题,deeper model。
2.3.2 相关模型
QnA Miner:基于半结构化和非结构化数据FAQ挖掘流程

百度搜索排序框架,基于深度学习来计算query和文本语义关联。
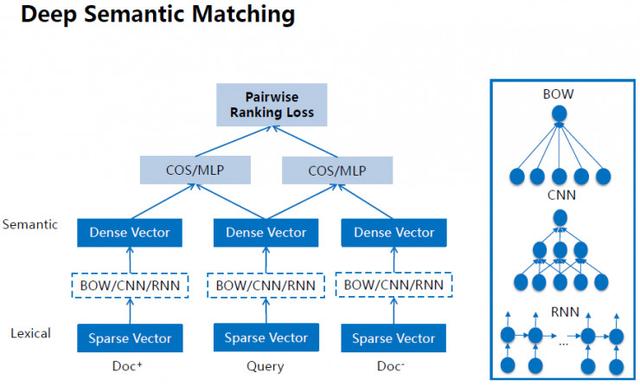
Convolutional Neural Tensor Network Architecture for Community-based Question Answering:基于CNN端到端的问题和答案匹配模型。

2.4 基于无结构文档的问答
2.4.1 主要问题
如何从冗余信息中找到与问题相关的片段,进而返回准确的答案。
- Attention-over-attention
2.4.2 相关模型
Attention-over-attention neural networks for reading comprehension:从文档中寻找问题的答案,采用了Attention-over-attention的机制。
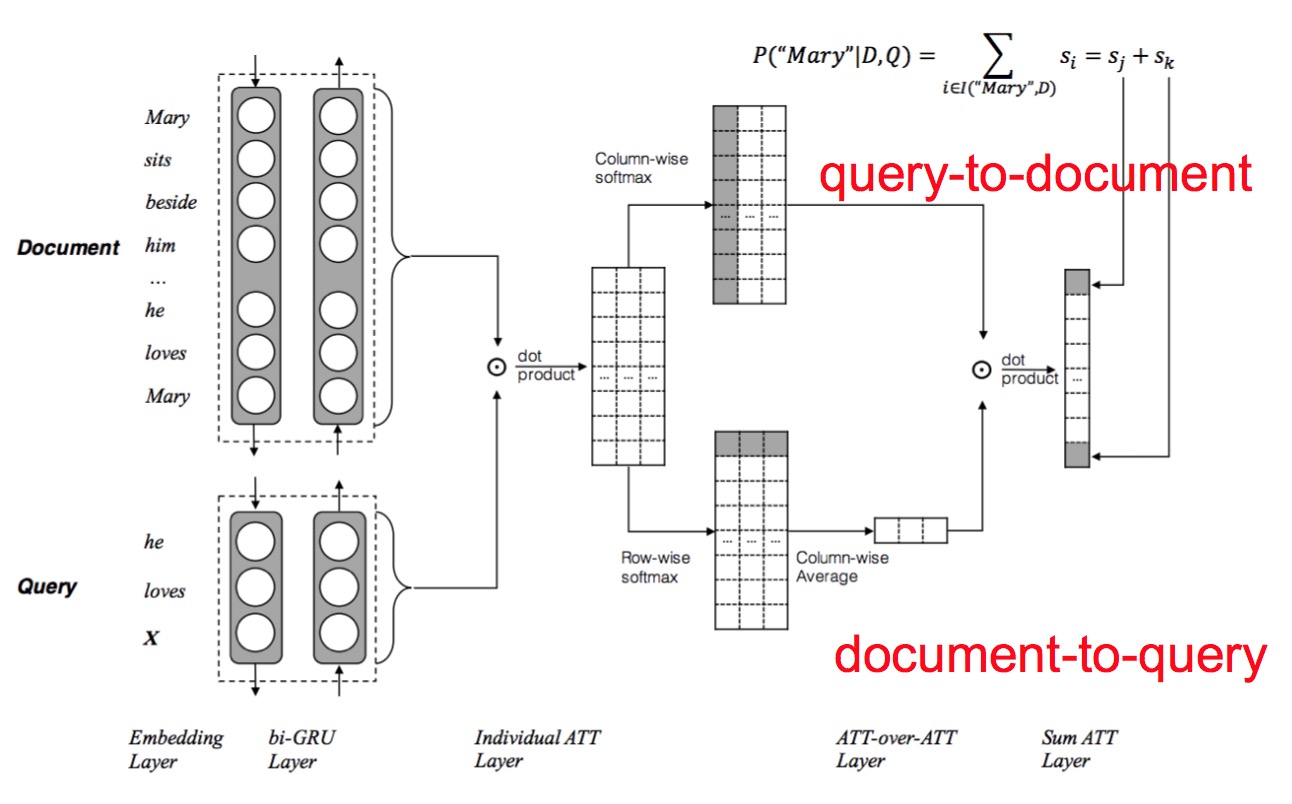
2.5 基于事实的推理问答
2.5.1 主要问题
如何基于自然语言的事实与问题进行推理建模。
- 外部记忆,分步进行推理
2.5.2 相关模型
Memory Network
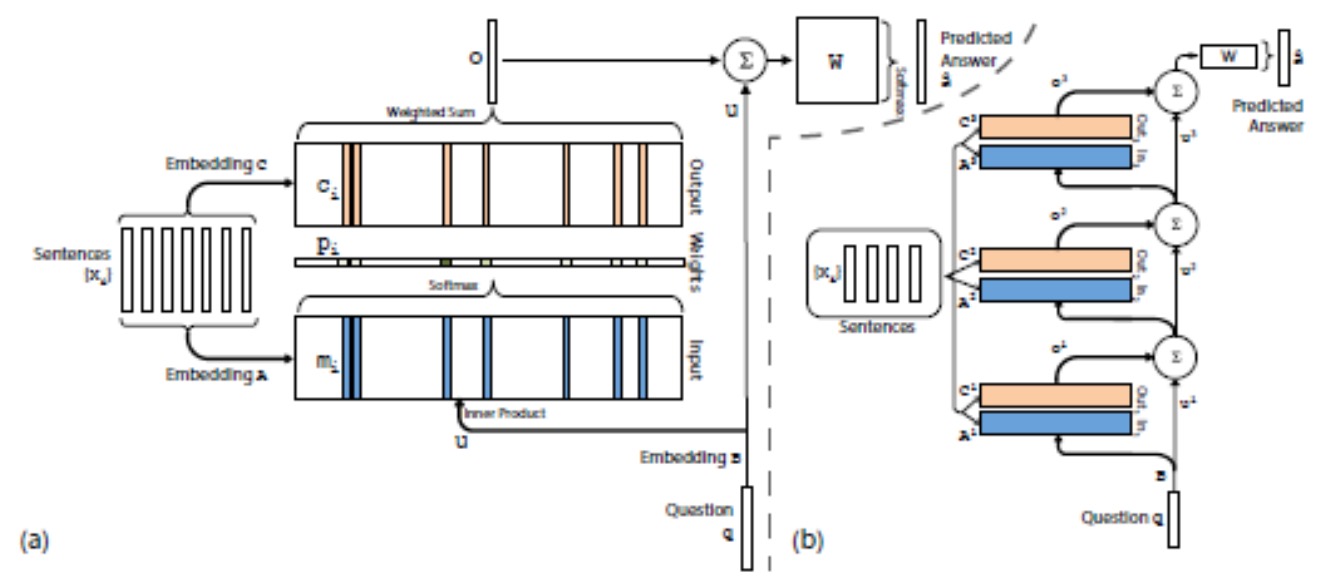
Dynamic Memory Network

Towards Neural Network-based Reasoning

3. 其他问题
如何将基于不同数据的问答系统进行整合
- 相对而言是一个工程方面的问题。
如何解决知之为知之不知为不知
- Robust Question Answering
- AI systems must produce accurate confidence values. Should “abstain” when they are uncertain
- AI systems should explain their reasoning
- AI systems should be robust to incorrect design assumption
- We need verification and validation methodologies for AI systems
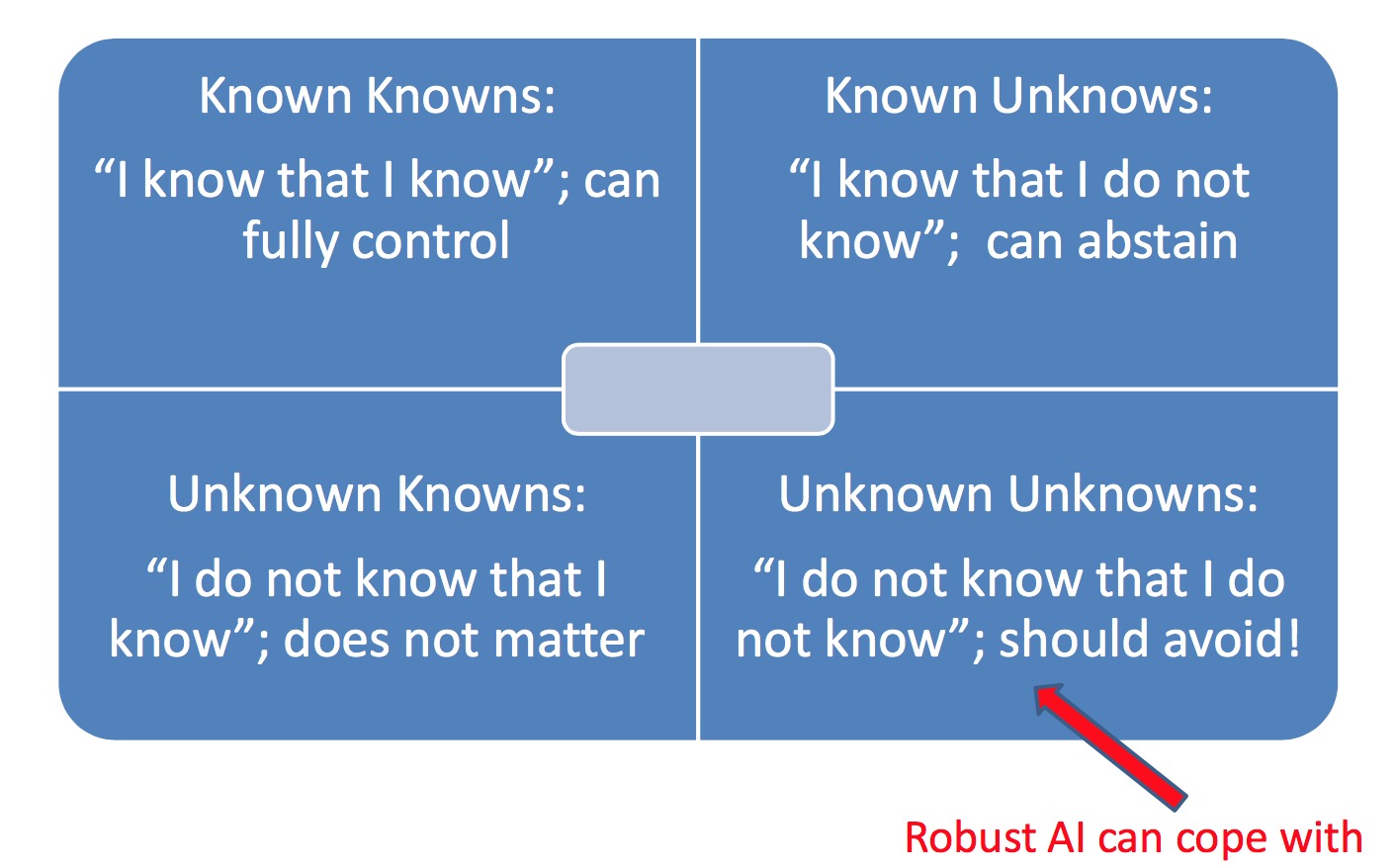
4. 引用
王海峰老师在AAAI 2017的报告
李磊老师在将门的报告
肖仰华老师在将门的报告
李航老师在AIRS 2016的报告
Yan Jun老师在知识图谱研讨会的报告
Recent Progress in Deep Learning for Natural Language Processing
Zhengdong Lu老师的报告